아웃라이어(Outlier)는 통계학, 데이터 분석, 그리고 다양한 사회적 맥락에서 사용하는 용어로, 전체 데이터 집합에서 다른 값들과 현저히 차이가 나는 데이터 점(data point)을 의미합니다. 쉽게 말해, "평균적인 경향에서 벗어난 이상치"입니다. 이러한 아웃라이어는 데이터 분석에서 매우 중요한 의미를 가질 수 있습니다. 때로는 분석 과정에서 제거해야 할 노이즈로 간주되지만, 특정 상황에서는 중요한 정보를 내포하고 있어 분석의 핵심이 되기도 합니다.
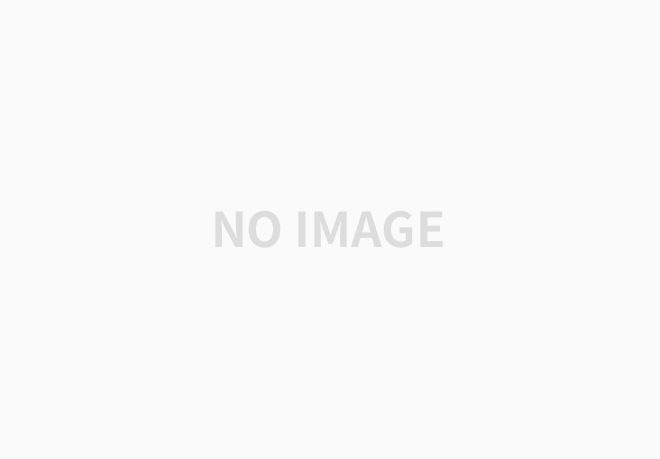
아웃라이어의 특징
- 평균과의 차이
대부분의 데이터 값들이 특정 범위에 몰려 있는 상황에서, 아웃라이어는 그 범위를 벗어난 극단적인 값을 가집니다. - 특정 상황에서의 유용성
- 문제 진단: 데이터 오류, 시스템 고장 등 비정상적인 현상을 나타낼 수 있습니다.
- 새로운 발견: 일반적인 패턴과 다른 중요한 통찰을 제공하기도 합니다.
아웃라이어의 종류
- 유형에 따른 분류
- 점 아웃라이어(Point Outlier): 단일 데이터 포인트가 극단적으로 다른 경우.
예: 학생 성적 데이터에서 특정 학생만 모든 과목에서 100점을 받은 경우. - 맥락적 아웃라이어(Contextual Outlier): 특정 맥락에서 비정상적으로 보이는 데이터.
예: 여름철에 눈이 내린다는 데이터. - 집합적 아웃라이어(Collective Outlier): 여러 데이터 포인트가 집단적으로 이상한 패턴을 형성하는 경우.
예: 금융 거래에서 특정 시간대에만 대규모 거래가 집중된 패턴.
- 점 아웃라이어(Point Outlier): 단일 데이터 포인트가 극단적으로 다른 경우.
- 원인에 따른 분류
- 측정 오류: 데이터 수집 과정에서 발생한 잘못된 값.
- 변화 또는 사건: 외부 요인에 의해 발생한 예외적인 데이터.
- 의도적 이상치: 사기, 공격 등 특정 목적에 의해 인위적으로 만들어진 데이터.
아웃라이어 식별 방법
1. 통계적 방법
- 평균과 표준편차: 데이터가 평균에서 특정 표준편차 범위를 벗어날 경우 아웃라이어로 간주.
예: ±3σ 범위를 벗어난 값. - 사분위수 범위(IQR):
- 1사분위(Q1)와 3사분위(Q3)의 간격을 기준으로 아웃라이어를 식별.
- 공식: IQR=Q3−Q1\text{IQR} = Q3 - Q1
- 아웃라이어: x<Q1−1.5⋅IQRx < Q1 - 1.5 \cdot IQR 또는 x>Q3+1.5⋅IQRx > Q3 + 1.5 \cdot IQR
2. 그래프를 활용한 방법
- 박스플롯(Box Plot): 이상치를 시각적으로 보여줌.
- 산점도(Scatter Plot): 데이터 간의 관계를 시각화해 극단적인 값을 파악.
3. 기계 학습 방법
- 클러스터링 알고리즘: K-means, DBSCAN 등을 사용해 정상 데이터와 분리.
- 이상치 탐지 모델: Isolation Forest, Autoencoder 등.
아웃라이어의 영향
긍정적 영향
- 새로운 발견의 기회 제공: 기존 데이터 패턴과 다른 새로운 인사이트를 제공.
예: 금융 사기 탐지, 질병 조기 발견. - 시스템 성능 개선: 데이터 오류나 문제점을 조기에 파악해 시스템 안정성을 높임.
부정적 영향
- 결과 왜곡: 아웃라이어가 포함되면 평균, 분산 등의 통계값이 왜곡될 수 있음.
- 모델 학습에 악영향: 기계 학습 모델의 성능을 저하시킬 가능성.
아웃라이어의 처리 방법
- 제거
- 데이터 오류로 간주되는 아웃라이어는 분석에서 제외.
- 예: 잘못된 입력값, 기기 오작동 데이터.
- 변환 또는 조정
- 로그 변환, 스케일링 등으로 극단적인 값의 영향을 완화.
- 개별 분석
- 특정 아웃라이어가 중요한 의미를 가질 경우 해당 데이터에 집중적으로 분석.
사례로 보는 아웃라이어
- 금융 사기 탐지
- 신용 카드 거래에서 비정상적으로 큰 금액이나 빈번한 거래를 아웃라이어로 간주.
- 의학적 진단
- 환자 데이터에서 정상 범위를 벗어난 특정 수치가 질병의 조기 경고 신호일 수 있음.
- 스포츠 기록
- 특정 선수가 다른 선수들에 비해 유독 높은 성적을 기록하는 경우.
아웃라이어와 관련된 주요 도서 및 참고
- 《아웃라이어》(말콤 글래드웰)
이 책에서는 성공한 사람들(아웃라이어)의 사례를 분석하며, 그들이 특출난 성과를 내기까지 어떤 환경적 요인과 노력이 있었는지 탐구합니다.
What is an Outlier?
An Outlier is a term used in statistics, data analysis, and various social contexts to describe a data point significantly different from other values in a dataset. In simpler terms, it refers to values that deviate markedly from the average trend, or "exceptional cases." Outliers can be crucial in data analysis, sometimes regarded as noise to be removed, while in other cases, they hold vital information and can become the focus of analysis.
Characteristics of Outliers
Difference from the Average:
Most data points cluster within a certain range, but outliers fall outside this range with extreme values.
Utility in Specific Situations:
- Problem Diagnosis: Indicate anomalies like data errors or system failures.
- New Discoveries: Provide insights that differ from general patterns.
Types of Outliers
By Type:
- Point Outlier: A single data point significantly different from others.
- Example: A student scoring 100 in all subjects while others score average marks.
- Contextual Outlier: Data that appears abnormal in a specific context.
- Example: Snowfall during summer.
- Collective Outlier: A group of data points forming an unusual pattern.
- Example: A large volume of transactions concentrated at a specific time in financial trading.
By Cause:
- Measurement Error: Incorrect values arising from data collection mistakes.
- Change or Event: Exceptional data due to external factors.
- Intentional Anomalies: Data deliberately altered for fraud, attacks, etc.
Identifying Outliers
1. Statistical Methods:
- Mean and Standard Deviation: Data points falling outside a specific standard deviation range from the mean are considered outliers.
- Example: Values outside the ±3σ range.
- Interquartile Range (IQR):
- Identify outliers based on the range between the first quartile (Q1) and third quartile (Q3).
- Formula: IQR=Q3−Q1IQR = Q3 - Q1
- Outlier: x<Q1−1.5×IQRx < Q1 - 1.5 \times IQR or x>Q3+1.5×IQRx > Q3 + 1.5 \times IQR
2. Graphical Methods:
- Box Plot: Visually depicts outliers.
- Scatter Plot: Visualizes relationships between data points to identify extreme values.
3. Machine Learning Methods:
- Clustering Algorithms: Use algorithms like K-means, DBSCAN to separate normal data.
- Anomaly Detection Models: Use models like Isolation Forest, Autoencoder.
Impact of Outliers
Positive Impact:
- Opportunity for New Discoveries: Provides new insights different from existing data patterns.
- Examples: Detecting financial fraud, early disease diagnosis.
- Improved System Performance: Early identification of data errors or issues enhances system stability.
Negative Impact:
- Distortion of Results: Outliers can skew statistics like mean and variance.
- Adverse Impact on Model Training: Can reduce the performance of machine learning models.
Handling Outliers
Removal:
- Exclude outliers considered data errors.
- Examples: Incorrect input values, device malfunction data.
Transformation or Adjustment:
- Mitigate the impact of extreme values through log transformation, scaling, etc.
Individual Analysis:
- Focus analysis on specific outliers with significant importance.
Case Studies Involving Outliers
Financial Fraud Detection:
- Identify abnormally large amounts or frequent transactions in credit card data as outliers.
Medical Diagnosis:
- Specific out-of-range values in patient data can be early warning signs of disease.
Sports Records:
- When an athlete consistently performs significantly better than peers.
Key Books and References on Outliers
- Outliers by Malcolm Gladwell: Analyzes cases of successful individuals (outliers) and explores the environmental factors and efforts contributing to their exceptional achievements.